- Jump Around
- Individuals Interviewed
In the shifting landscape of value-based care, we believe one tool has emerged as essential: risk stratification models. These algorithms are becoming the backbone of modern care organizations, transforming healthcare from a reactive, fee-for-service treadmill into a proactive system that identifies and manages high-risk patients before they spiral into costly medical crises.
From a financial perspective, the stakes couldn’t be higher. A well-calibrated risk model, when paired with next-best-action clinical interventions, has the potential to bend the cost curve, reducing unnecessary hospitalizations and lowering overall medical spend. But the real question is: can AI push these models beyond their traditional limitations? Can it predict severity and future spend with a level of precision that old, rules-based systems never could?
One emerging player betting big on that premise is Delorean AI—a company that has drawn interest from multiple Rubicon portfolio ventures. Their approach blends rules-based engines with black-box AI models, a hybrid strategy designed to extract deeper insights from complex patient data.
What sets Delorean apart is the scale of its training ground. Their models were honed on a massive dataset of 40 million claims from UnitedHealthcare, and the company isn’t shy about making bold claims: 80-90% predictive accuracy compared to 60% from legacy Optum models 1.
Delorean’s strategy isn’t just about broad predictive power—it’s targeted. They offer off-the-shelf risk stratification models focused on high-impact disease states: renal, diabetes, cardiovascular, mental health, and respiratory conditions. The goal? To move beyond static risk scores and into real-time, AI-driven forecasting—an intelligence layer that could revolutionize how healthcare systems anticipate and prevent deterioration.
But as with any black-box AI model, the question remains: can it be trusted? Accuracy claims are one thing—proving real-world impact is another. If successful in proving out the model, Delorean is positioned to set the pace in this important segment.
“Our Real-Time and Future Risk Stratification is a first in the healthcare industry. It can not only assess a patient’s risk in real time but also predict future risks—what is the delta and how to reduce these scores. We are essentially the Future for Healthcare,” says Severance MacLaughlin, CEO and Co-Founder, Delorean AI.
The Delorean Interface flags patients in need of likely interventions to get ahead of MLR expenditures
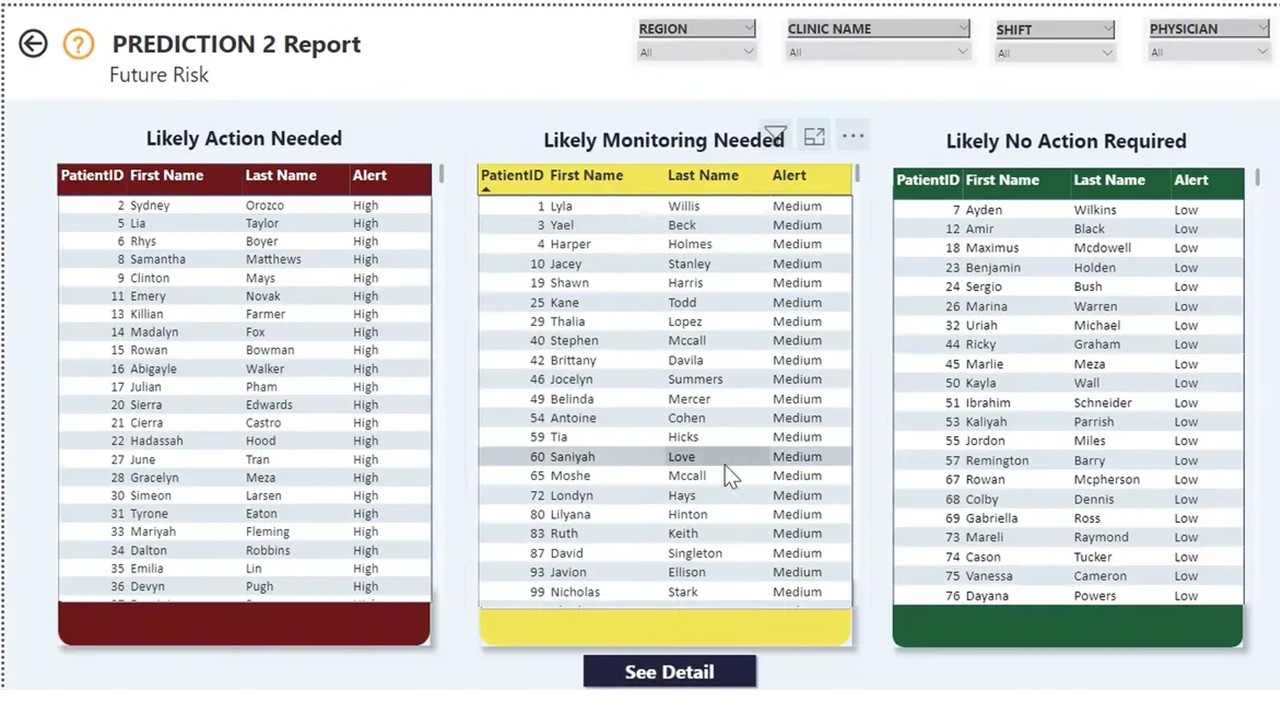
Note from Delorean AI: The content used in the slide above is synthetic data
“The use of AI for risk stratification in the renal use case is promising but we still need to see additional improvement before this tech is deployment ready,” said Ron Margalit, Chief Information Officer at Evergreen Nephrology.
In the delicate world of hospice care, timing is everything. The ability to anticipate a patient’s decline—not just in broad clinical terms, but with granular, data-driven precision—can mean the difference between a well-managed, dignified transition and a crisis that leaves families and caregivers scrambling.
Medalogix Muse, an AI-powered platform, is quietly reshaping how hospice providers assess patient risk. Unlike traditional models that rely on structured medical data, Muse taps into unstructured EMR data, extracting signals from clinician notes, assessments, and patient histories to calculate risk scores for transition risk, mortality risk, and overall patient risk.
What sets Medalogix apart is its deep integration with Homecare Homebase (HCHB)—the dominant EMR system in the hospice sector. This tight coupling allows the platform to seamlessly ingest raw, unstructured data, pass it through natural language processing (NLP) and machine learning algorithms, and surface actionable insights for hospice teams.
The result? A population health dashboard that lets providers sort and stratify patients by risk level, helping them prioritize care for those most in need. In an industry where staffing shortages and unpredictable disease trajectories make resource allocation a constant challenge, having an AI-driven roadmap for patient decline is a powerful proposition.
Medalogix claims substantial penetration within the HCHB user base 2, a sign that hospice providers are increasingly willing to lean on AI in what is, arguably, one of the most sensitive and human-centered branches of medicine. But the real test lies ahead: can AI-driven risk stratification truly improve end-of-life care, or will it become just another layer of prediction in a field that ultimately defies perfect foresight?
The Muse Interface offers individual and population-level views of patient risk in the Hospice setting.
Muse offers an individual deep dive on predictive metrics in a hospice setting
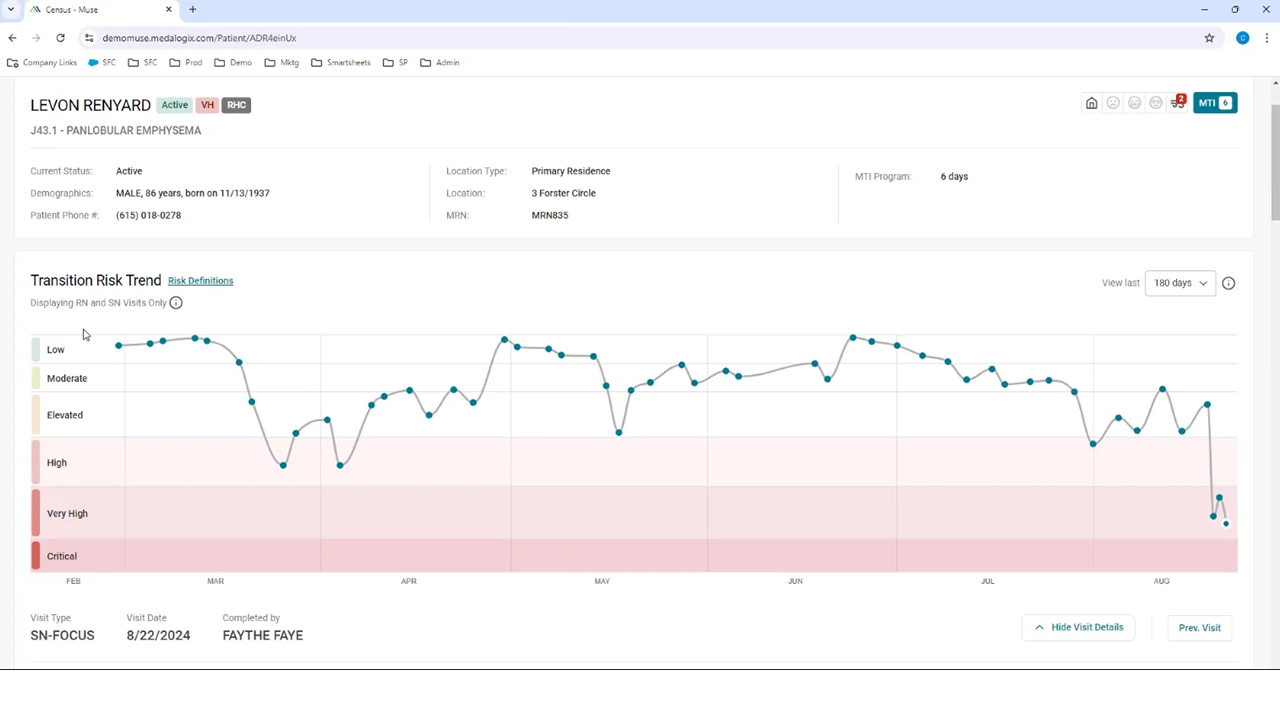
Note from Medalogix: The content used in the slide above is synthetic data for illustrative purposes
Muse also offers a more aggregated view of the patient panel
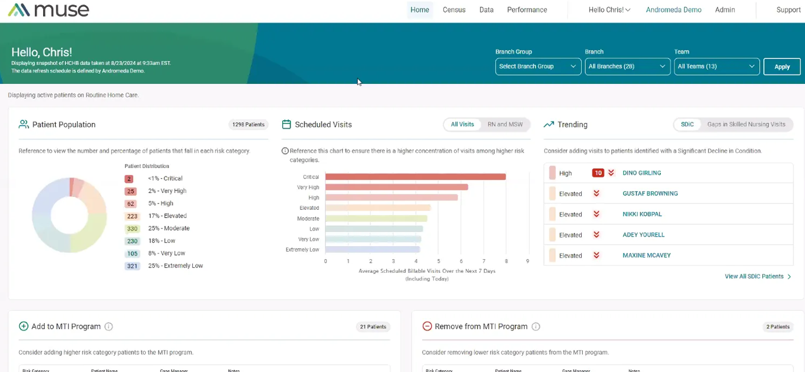
Note from Medalogix: The content used in the slide above is synthetic data for illustrative purposes
For Imagine Pediatrics, a company dedicated to caring for children with complex medical and behavioral health needs, the traditional and emerging risk stratification models simply weren’t enough. They needed something faster, smarter, and more precise—a system that could move beyond the lagging indicators of the past and provide real-time, actionable insights for a population where every moment counts.
In most of the industry, risk stratification has long been claims-driven. It’s a familiar approach—using insurance claims data to assess patient risk—but we believe it comes with a fatal flaw: time lag. Claims data can be weeks or even months old, leaving providers reacting to problems instead of anticipating them. Instead of relying solely on claims, Imagine built a model that integrates real-time data from multiple sources: their EMR, Health Information Exchanges (HIEs), additional rich data streams, and their own proprietary insights. This multilayered approach allows them to capture a more dynamic and accurate picture of patient risk, giving providers early warning signals rather than delayed snapshots.
But the potential breakthrough is in how this data is applied. Most risk models distill patients down to a single score—a number that signals risk level but offers little in terms of tailored care. Imagine Pediatrics has taken a different approach. Instead of assigning a static risk score, they segment patients into cohorts based on a highly individualized model of medical and behavioral pathways.
These cohorts are actionable, meaning they’re directly linked to specific interventions, personalized treatment plans, and targeted care journeys. For children with special health care needs, this level of precision isn’t just useful—it’s game-changing. It allows for more timely, more effective, and more personalized interventions, ensuring that the right resources reach the right patients at the right time.
“Our unique approach to risk stratification and the leveraging of real time data is a game changer for us because our patients have unique, individualized needs and are quite vulnerable at discrete times, including the moment of discharge from an acute setting. The combination of rich data sets and input from our multidisciplinary, pediatrician-led clinical team, enables us to generate insights on the right type of care each patient needs at any point in time and drives clinical teams to next best actions,” said Eden Klein, CTO at Imagine Pediatrics.
The Imagine Pediatrics Model: To go beyond simple risk stratification to actionable patient cohorts
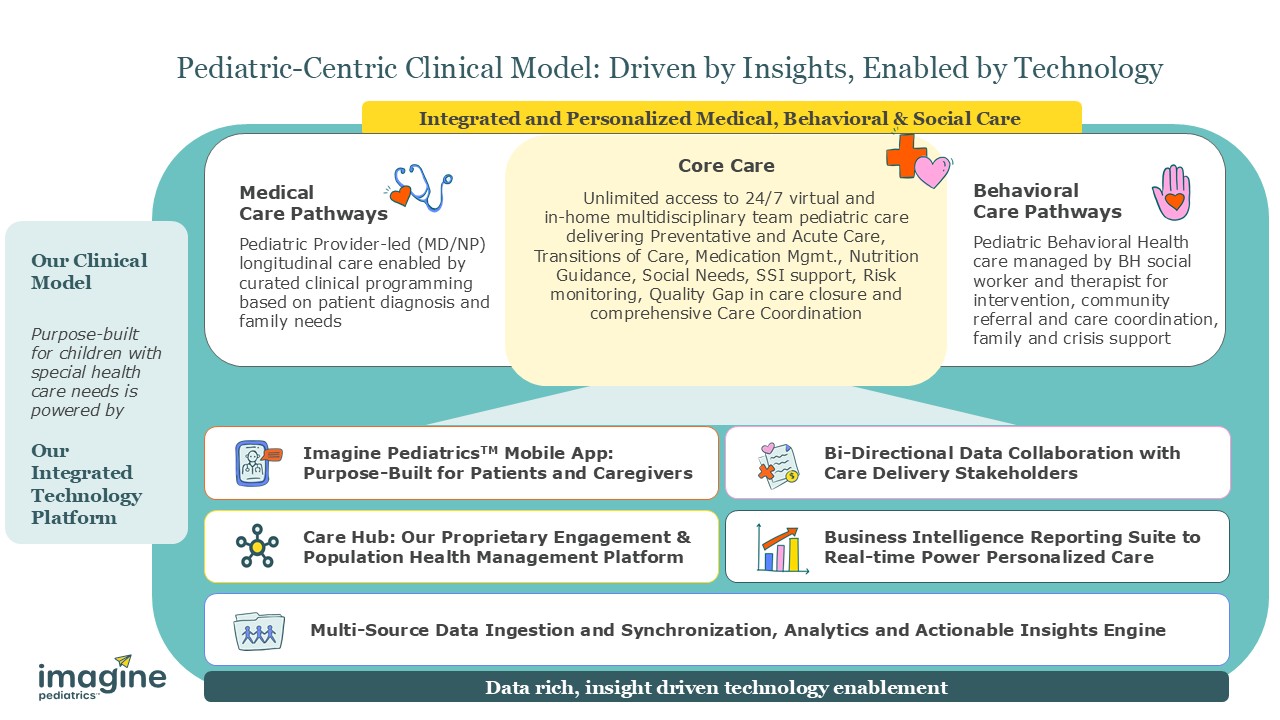
Despite some success in this area, many healthcare leaders continue to voice skepticism based on historical experiences with risk stratification models:
“There continues to be a paucity of risk stratification algorithms that can actually drive the care team to a good next best action. It makes me wonder if the risk stratification algorithmic market is a commodity market with little differentiation. It has to turn into work that matters,” said Bartley Bryt, Chief Medical Officer at Privia Health.
“Part of the problem is the experience specialists have had to date with more immature models. Risk Stratification algorithms that serve up specific diagnostic code recommendations on patients can quickly lose credibility if those codes are a total mismatch with the patient type (i.e. diabetic codes for a non-diabetic),” says Pflederer, Chief Medical Officer, Evergreen Nephrology.
Overall, we see a need for a new generation of risk stratification - “Risk Stratification 2.0” - that uses an approach like the one deployed by Imagine Pediatrics to address some of these concerns.
Technology could help make Risk Stratification more actionable
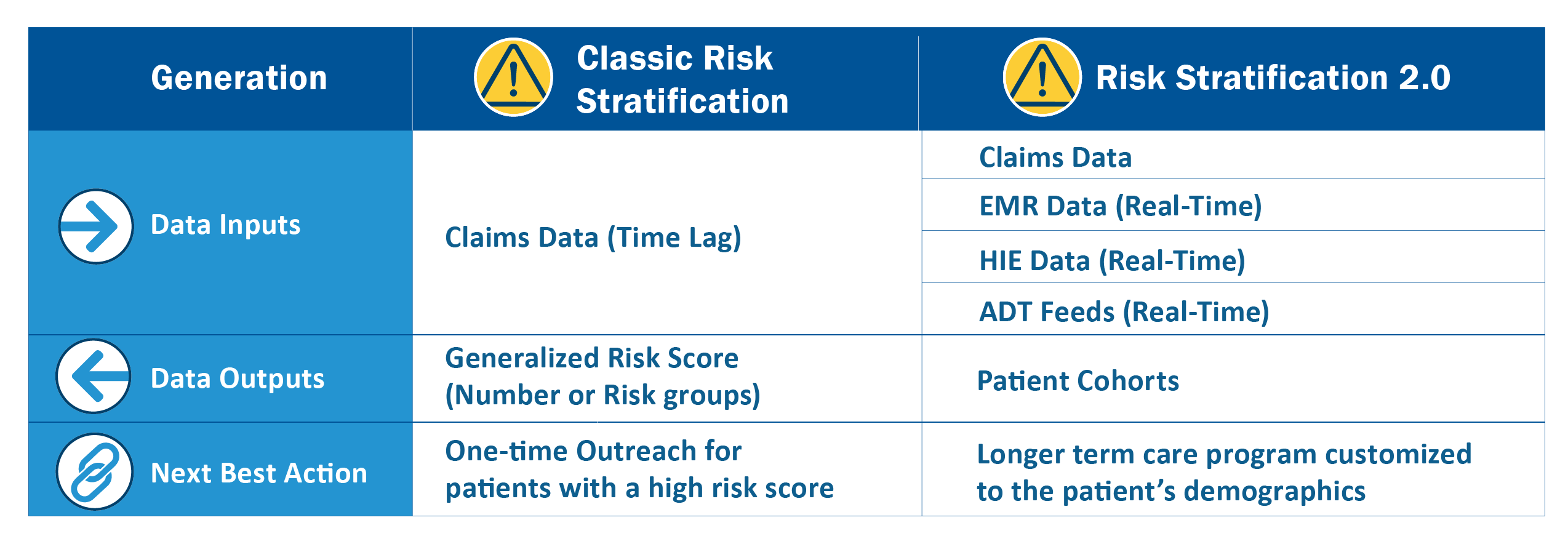
With the integration of broader real-time data sets, actionable risk stratification may be more attainable for value-based care organizations.
Parting Thoughts
AI is often framed as a clinical revolution, a toolset that will transform diagnostics, treatment plans, and patient engagement. But beneath that lofty promise lies an equally compelling, if less glamorous, reality: AI’s potential to reshape the financial picture of healthcare services.
We believe early financial models suggest modest AI-driven efficiencies can unlock significant EBITDA growth. And that financial health—often overlooked in conversations about AI—directly translates to mission sustainability. A more profitable healthcare organization is one that can invest more in patient care, expand services, and improve outcomes.
Across our portfolio, AI innovation is in full swing. Categories like Ambient Scribing and Risk Stratification are seeing rapid adoption, though some markets—especially Ambient Scribing—are becoming crowded.
Yet, not every healthcare AI use case lends itself to black-box machine learning models. In domains where explainability and transparency matter, rules-based engines still have a role to play—and they are unlikely to vanish. Algorithmic identifier-driven business models serve as a prime example: where precision matters, buyers are reluctant to trust opaque AI outputs without human oversight. That’s why many organizations are deploying human-in-the-loop AI, a strategy that balances automation with clinician judgment. Looking ahead, we’ll continue to explore the evolving landscape of AI in healthcare, with a focus on:
- Population health software and insights from Rubicon companies working with vendors like Zus and Innovaccer.
- The rise of back-office automation tools like Tennr and voice AI agents like Hippocratic AI—and how agentic AI models could redefine efficiency in healthcare.
The AI revolution in healthcare is far from settled. The question isn’t whether AI will reshape the industry—it’s which models, which approaches, and which business strategies will prove most enduring, and we look forward to exploring it all with you here.
Disclaimer: The use of AI in healthcare is nascent and evolving rapidly. Views expressed in this paper are based on preliminary findings and analysis. The long-term benefit of the impact of AI in both the healthcare industry and any investments made by Rubicon Founders will be determined over many years or decades. Additionally, any real impacts will likely occur after much research and development time and cost, and not all projects will achieve positive return on investment.
Individuals Interviewed for this Article
Rubicon Portfolio Companies
Jason Foucher, Chief Product Officer, Indigo
Eden Klein, Chief Technology Officer, Imagine Pediatrics
Meghan Haycraft, Co-Founder & Chief Strategy Officer, Imagine Pediatrics
Sonnie Linebarger, CEO, Cadre Hospice
Ron Margalit, Chief Information Officer, Evergreen Nephrology
Tim Pflederer, Chief Medical Officer, Evergreen Nephrology
Joe Lucero, Vice President of Risk Adjustment, Honest Health
Jeffrey Stevens, Chief Medical Information Officer, HarmonyCares
Bartley Bryt, Chief Medical Officer, Privia Health
Irfan Ali, Chief Information Officer, US Heart and Vascular
AI Technology Vendors
Fawad Butt, CEO, Penguin Ai
Ronen Lavi, CEO, Navina
Jay Ackerman, CEO, Reveleer
Michael Ng, Co-Founder and CEO, Ambience Healthcare
Sandeep Gupta, Co-Founder and COO, Innovaccer
Severence MacLaughlin, CEO and Co-Founder, Delorean AI
Punit Singh Soni, Founder and CEO, Suki